AI has made its way into mainstream culture and changed how we look at the world going forward. One such industry is healthcare — where AI in healthcare discussion opens up numerous opportunities.
The AI industry is booming and is expected to reach around 188 billion US dollars by 2030. It will help solve several healthcare issues, such as preventing diseases, discovering and developing drugs, and diagnosing treatments.
These AI solutions help streamline operational costs, increase efficiency, and improve patient outcomes. But that’s not all.
Let’s learn how AI can be used in healthcare, its benefits, and how startups can benefit from AI.
Importance of AI in Healthcare
Advanced technologies like AI are already reshaping how medical experts deal with patient care, diagnosis, potential treatments, and more.
Not only this, if AI is extensively integrated with healthcare, it has the potential to promote savings of about $360 billion a year. This trajectory shows AI won’t only benefit in terms of promoting patient health or diagnosing diseases faster – it’ll also promote economic advantages.
Currently, AI focuses on diagnostic techniques that assist physicians in decrypting medical images like resonance imaging, X-rays, and MRI scans, resulting in quicker and more accurate diagnoses.
Several healthcare sectors also heavily rely on AI techniques like diagnostic imaging and computer vision, natural language processing (NLP), and clinical decision support systems (CDSS). Computer vision helps to propel the process of medical imaging interpretation, NLP helps extract and understand information from medical texts, and CDSS assists healthcare practitioners in making informed decisions based on patient data and medical records.
These help medical practitioners go the extra mile and uncover historic unseen correlations in healthcare data, such as detecting subtle changes that may indicate a potential problem.
Need help implementing AI into your project? At Iterators we can help design, build, and maintain custom software solutions for both startups and enterprise businesses.
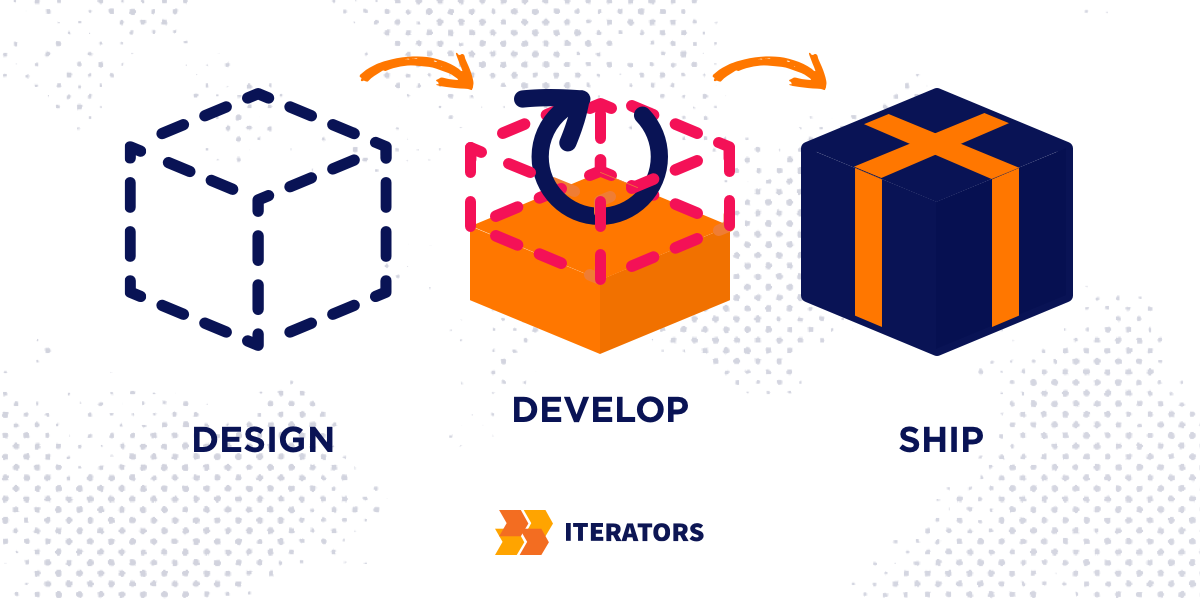
Schedule a free consultation with Iterators today. We’d be happy to help you find the right software solution to help your company.
Applications of AI in Healthcare
As a medical practitioner, your goal is to determine the cause of a problem and orchestrate a detailed medical evaluation. This involves taking various diagnostic tests, biopsy procedures, and blood tests. However, this takes time.
AI can make all these processes faster, helping you quickly determine the best course of patient treatment. Let’s look at some AI applications in healthcare and understand how they’re changing the industry.
1. Machine Learning
Machine learning includes models that fit certain data and learn by training models with data. These algorithms can process huge amounts of data, identify patterns, and accurately predict medical outcomes.
ML also enables you to accurately predict the prevention strategies and treatments that will support the different patients you deal with. It further branches out to several types, including:
a. Neural Networks
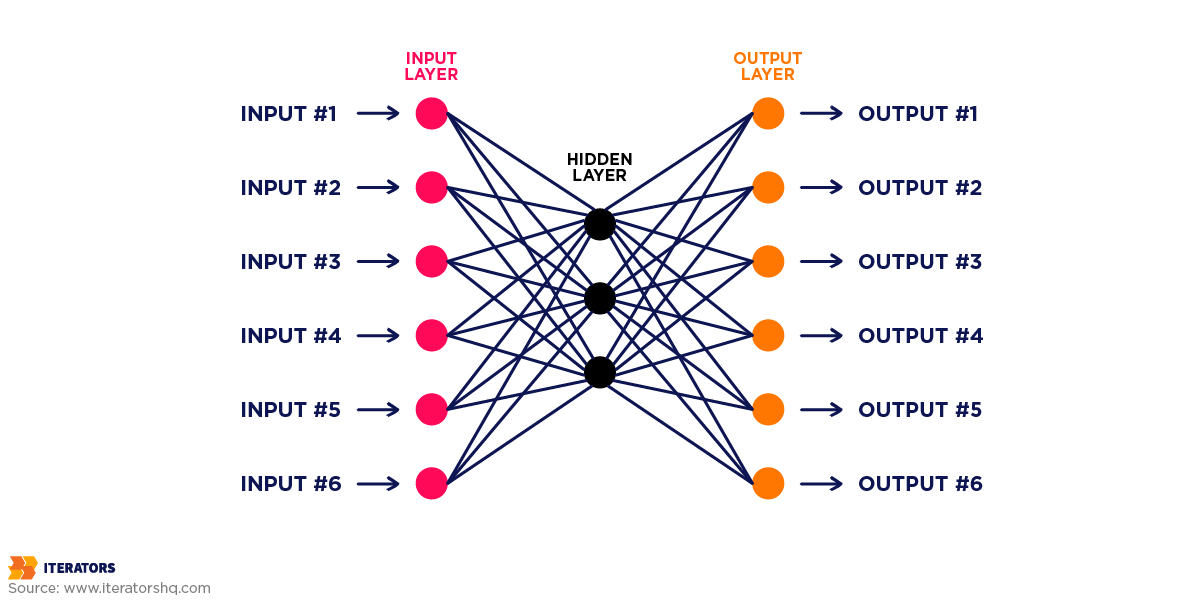
Neural networks are a subset of machine learning and serve as the building blocks for deep learning algorithms. They have been available since the 1960s and are widely used in applications like determining whether or not a patient can catch a potential disease.
These networks view the healthcare problems as inputs, outputs, and features associated with those inputs and outputs. They mimic the way neurons in the human brain process signals.
Studies show that machine learning models like neural networks are used in precision medicine. They help to see which treatment protocols will likely work on a patient based on different patient attributes and the treatment’s context.
b. Deep Learning
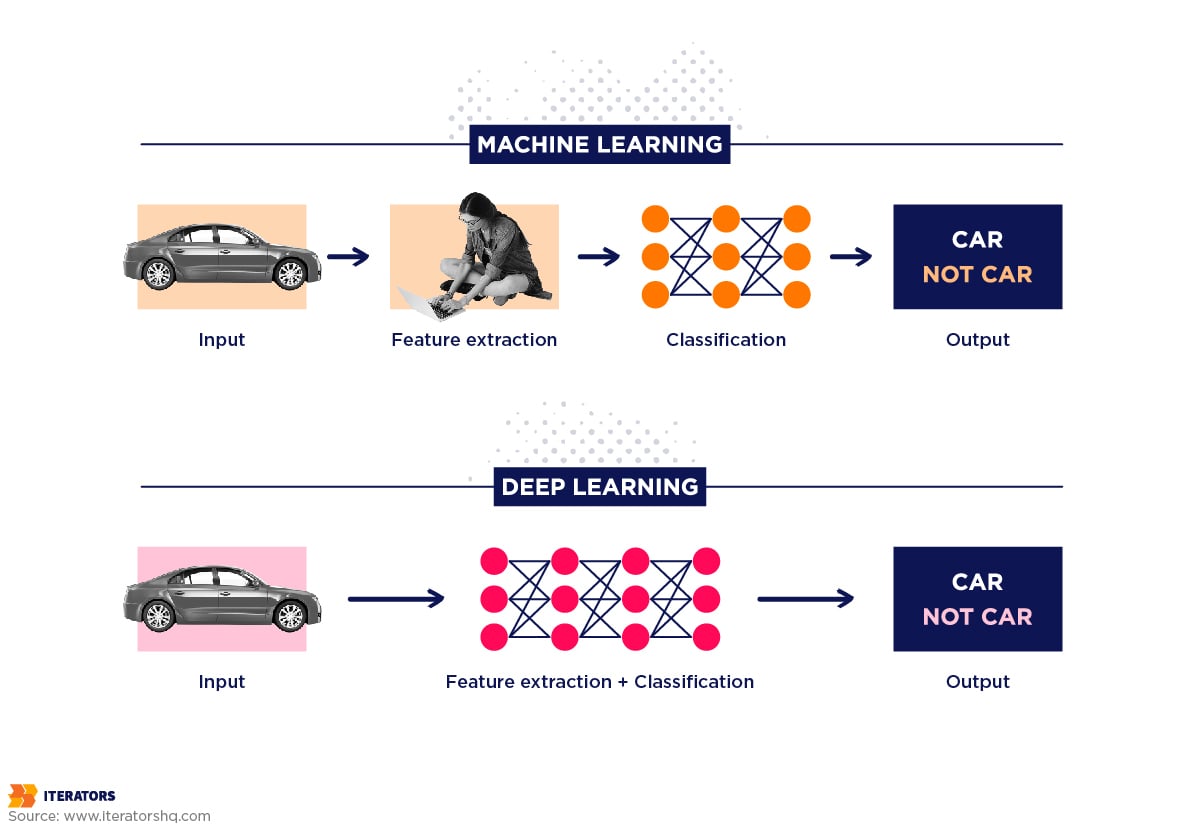
Deep learning (DL) is a complex form of machine learning with many layers of neural networks that predict outcomes. These networks contain thousands of attributes that can be quickly processed with the help of cloud architectures and graphics processing units.
DL models help analyze electronic health records (EHRs) that contain data like laboratory tests, clinical notes, and medications at extraordinary speeds and yield high accuracy.
A common application for DL algorithms in healthcare is recognizing potential cancerous lesions in radiology images. Deep learning is extensively being used in radionics or in clinical features in imaging data that can be perceived by the human eye.
2. Medical Imaging
Medical imaging is the different technologies used to look at the human body to monitor, diagnose, and treat medical conditions.
AI algorithms can be trained to detect brain tumors by checking MRI scans. They can also help to diagnose cerebrovascular diseases by analyzing CT images and recognize early-stage dementia and Alzheimer’s diseases by evaluating small changes in the brain.
Some other AI-based medical imaging use cases include:
- Analyzing various disease characteristics not detectable by the naked eye.
- Detecting image modalities at different treatment stages
- Identifying complex patterns in imaging data
- Evaluating radiographic traits in a quantitative manner
Moreover, AI-powered medical imaging improves the accuracy of medical diagnoses and helps predict diseases and initial patient screenings.
For instance, AI technologies help track patients’ conditions and even detect the smallest change in a large amount of data. This quality is beneficial for tracking brain tumors and other types of sensors. In comparison, traditional imaging techniques cannot evaluate the percentage of tumor cells that are alive or dead.
3. Rule-based Expert Systems
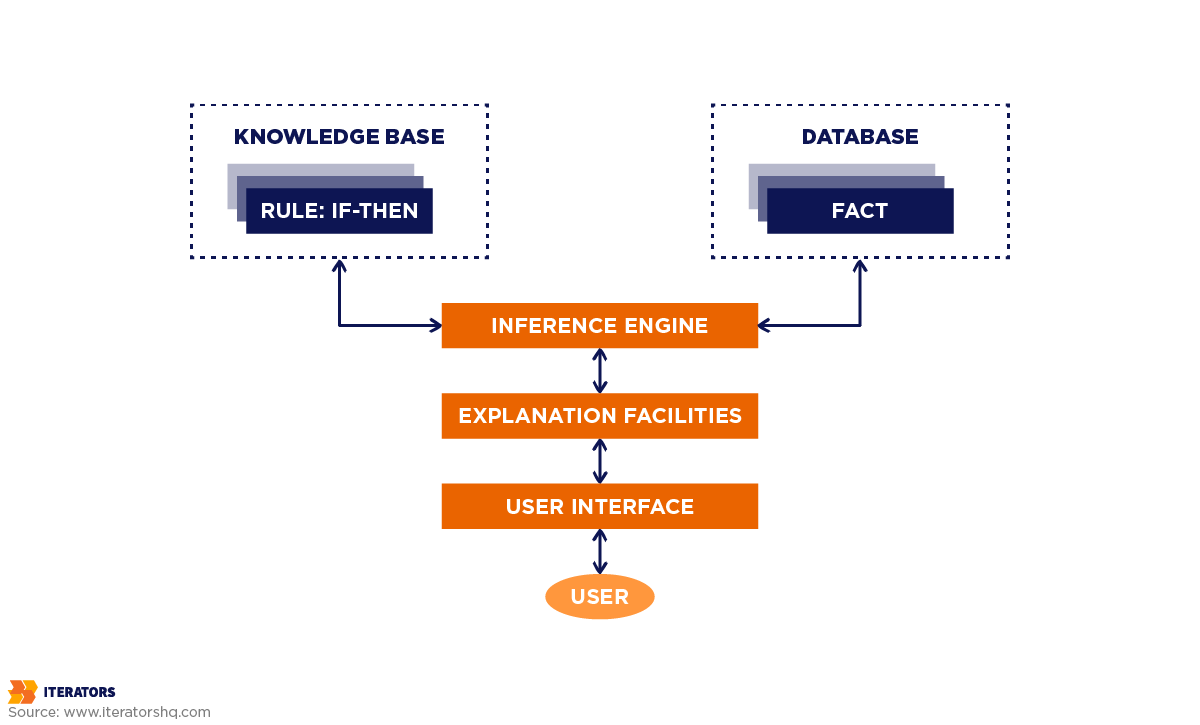
Rule-based expert systems include a set of “if-then” like statements (i.e., conditional statements) that use a set of assertions. They preserve and utilize knowledge that is encoded in the form of rules.
Each rule encodes a small piece of the expert’s knowledge and is divided into two sides. The main idea behind these systems is to use human expert knowledge in the real world through the help of computers.
In healthcare, these rule systems are widely used for clinical decision-support purposes. Many EHR (electronic health records) providers filter a set of rules for use in their systems.
Moreover, these expert systems only rely on humans and experts to build the set of rules included. The system works fine until a certain number of rules is inputted.
However, as more rules add up, the number can often exceed thousands of rules that go into conflict with each other and fall apart. So, machine learning helps replace rule-based systems by interpreting data and making it easier to change rules.
4. Natural Language Processing (NLP)
NLP is an AI technology that cleans a dataset. This means the input you’ll give to the computer will be organized into a more logical format — like breaking up the text into small semantic units, also known as tokens.
After that, it’ll use algorithms to interpret the text. This enables NLP to analyze huge amounts of patient records and provide incredible insights that improve medical methods.
Healthcare providers use NLP to help categorize the frequency, strength, duration, and form associated with a specific drug. This is called the clinical extraction model, achieved by making connections in entities the NLP algorithm detects. This helps support clinical documentation by looking at pertinent data for relationships between key phrases and words.
Patient Participation and Adherence
Research shows that less than 25% of patients stay highly engaged with their treatment plans. AI helps here by promoting deeper involvement from the patient’s side — providing relevant alerts and targeting content that can provoke the patient to take action and adhere to their treatment plans.
AI uses large datasets from different sources – these include consumer behavior data, medical claims data, social determinants of health, etc. It helps healthcare facilities make smarter and more strategic moves around patient engagement.
Moreover, AI leverages data-driven insights that help healthcare facilities focus on patient outreach and determine the timing and frequency of communications.
So, by using information from EHR systems, watches, smartphones, biosensors, and other data modes, AI can tailor recommendations to compare patient data and recommend tailored patient care.
Treatments and Diagnosis
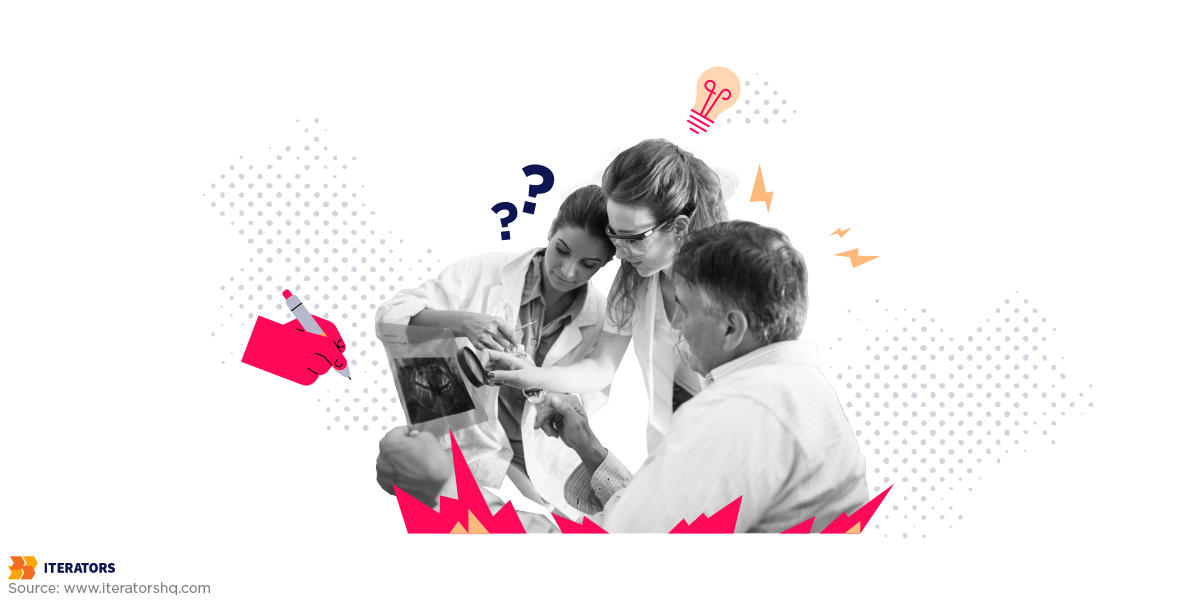
An AI algorithm is fed by thousands of data points to differentiate between health and disease patterns. The more training data it receives, the better it’ll learn and the more accurate results it will give. With each passing day, these algorithms become sophisticated enough to determine whether someone has a risk of developing chronic diseases.
For instance, in a study involving 521 participants at 10 centers for diabetic retinopathy diagnosis, an AI system showed higher sensitivity in detecting diabetic retinopathy compared to ophthalmologists. The system could be a cost-effective tool for diabetic eye screening.
Another example is with mammography. The majority of the AI algorithms show accurate results in breast cancer detection, with some models that have showcased similar or even more accurate results to those of radiologists.
Also, studies using deep-learning CAD – Computer Aided Detection – in mammography show significantly improved radiologists’ diagnostic performance. AI-CAD demonstrated superior performance in detecting various types of cancers, including masses, distortions, asymmetries, early-stage, node-negative invasive cancers, and those in mammographically dense breasts.
So, AI is already helping to go deeper into a standard 3D mammogram and identify risk patterns for breast cancer patients. It also helps negate the chance of false positives and is already playing an important role in the early detection of breast cancer.
Ethical Considerations When Using AI in Healthcare
Artificial intelligence in healthcare will challenge the existing status quo as it adapts to new technologies. This will result in a changed relationship between patient and provider.
This also paves the path for ethical considerations within the industry. Challenges like privacy and data protection, ethical dilemmas, social gaps, informed consent, medical consultation, sympathy, and empathy are just some of the considerations of AI.
So, before integrating AI completely with healthcare, healthcare professionals have to consider all four medical principles, including:
- Beneficence – Prioritizing actions that enhance the patient’s well-being.
- Nonmaleficence – Ensuring healthcare actions do not cause intentional harm.
- Autonomy – Respecting patients’ right to make decisions about their own healthcare.
- Justice – Ensuring fairness and equal distribution of healthcare resources.
Moreover, they also have to pay attention to the following:
1. Lack of Transparency
One of the most difficult issues to address here is transparency. If your patient is informed a single image has diagnosed cancer in them, they would want to know how and why.
Unfortunately, most AI algorithms, especially the ones that use deep learning for image analysis, are impossible to explain or interpret virtually. This is because AI algorithms operate as complex neural networks. These networks have interconnected layers – which make it challenging for you to understand how they arrived at a particular decision or prediction. 2. Preference of Human Interaction
There can also be cases where the patient wants no “robotic” intervention at all and would prefer to receive care from a clinician.
Plus, machine learning systems are also prone to algorithmic bias — they can predict greater likelihood of disease based on gender, race, or sex when those are not the actual variables. For instance, if the historical data that was used to train the machine learning model contains disparities, the model may learn to make predictions based on that learning data. The consequences are inefficient results that may not accurately represent the individuals health status or needs.
2. Autonomy and Informed Consent
Patients have the right to their health status, treatment process, diagnoses, test results, health insurance, cost, and any medical information related to them. Concerns about the lack of consent increase with the rise of AI in healthcare applications.
Using the autonomy principle in healthcare, we know that all patients have the right to ask questions about their treatment, be aware of the process, and refuse treatment even if the healthcare provider finds it the best solution.
Drug Discovery and Development with AI
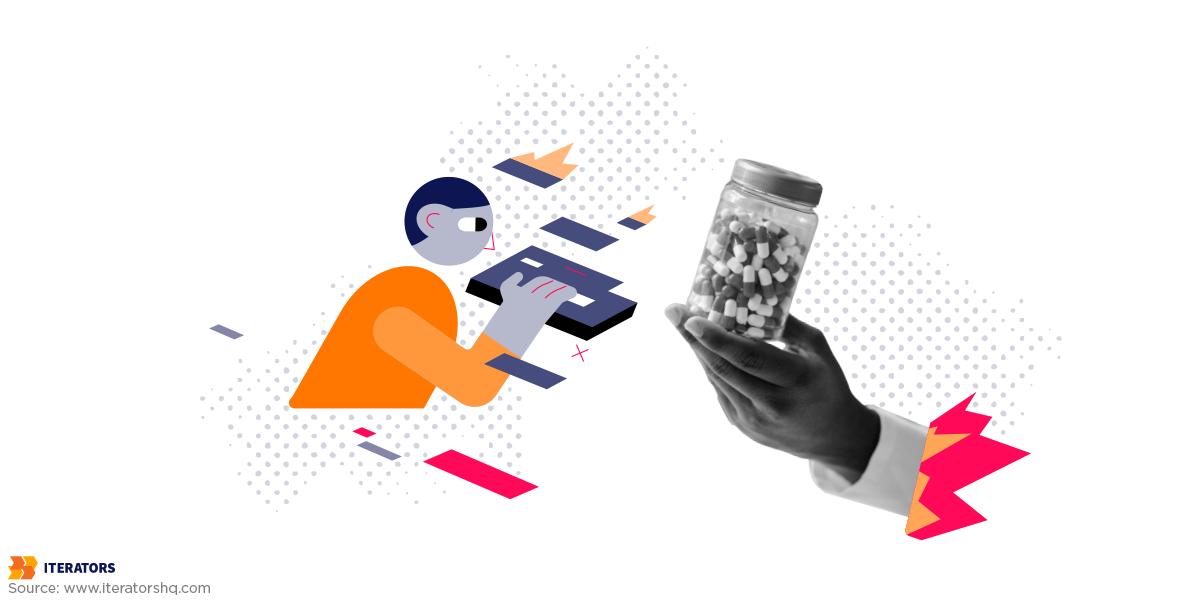
Conventionally, drug discovery requires years of laborious effort to develop complex new medications. It passes through intensive techniques like trial-and-error experimentations and high-throughput screening.
With AI, this process can be accelerated. Techniques like deep learning and NLP help to analyze large amounts of data accurately and predict a drug’s efficacy. However, the following also helps as well:
1. Drug Compound Optimization
AI helps with rational drug design, determines the right therapy for a patient, helps in decision-making, and helps with personalized medicines.
It assists in virtually screening and optimizing compounds and predicting protein-drug interactions. These models can be trained with data like molecular descriptors, protein-ligand complexes, and structural information of compounds.
2. Hit-and-Miss Approaches
At the moment, the medicine industry relies heavily on a hit-and-miss approach. This involves evaluating large numbers of potential compounds. The problem with conventional medicine is that these methods are costly, slow, and give low-accuracy results.
Since AI is based on different algorithms, including unsupervised and supervised learning methods, rule-based algorithms, or reinforcement ones, it can reduce these problems.
For example, the toxicity and efficacy of different drug elements can be predicted with AI algorithms — which ensures greater accuracy and efficiency than traditional testing.
3. New Drugs
An AI-based drug development team can spot new targets to develop drugs, like genetic pathways or specific proteins.
Drug discovery is a notorious process – it can spend 3-6 years in only its pre-clinical stages and cost hundreds of millions to billions of dollars. In the initial stages, AI can be used to train large datasets and help the models to understand biological mechanisms of diseases that can be targeted to counteract.
Plus, AI is also being used to limit requirements for physical testing of a drug compound by using high-fidelity molecular simulations that can run entirely on computers.
Limitations of Using AI in Drug Development
Although AI paves the path for efficient development, it still has several limitations, such as:
1. Insufficient Data for Drug Development
One of the challenges is the availability of adequate data. Since AI algorithms require a significant amount of data to train their algorithms, a lack of suitable data can cause several anomalies in the results due to inconsistencies and low-quality data.
2. Ethical Challenges
Another challenge is the ethical considerations. There’s much debate regarding the bias and fairness of AI-based algorithms. For instance, if the training data is unfair or only accounts for a certain gender or demographic, the outcome predictions can be inaccurate.
One of the AI solutions to this problem in healthcare is the use of data augmentation. This technique increases the diversity of your training set by using synthetic data to existing datasets.
It also helps to improve the diversity of the data for training algorithms, which ultimately improves the reliability and accuracy of the outcomes.
Another approach is the AL (XAI) method. This provides transparent explanations for predictions presented by AI algorithms. The solutions help to address the bias and lack of fairness aspect in AI-based solutions by providing a deeper understanding of the reasons behind each prediction.
Nonetheless, AI-based solutions aren’t ready to replace traditional research. Thus, they can’t take the place of human experts and experience. These models are only suitable for predictions based on available data. Even then, the results have to be evaluated by human researchers.
Healthcare Professionals and AI
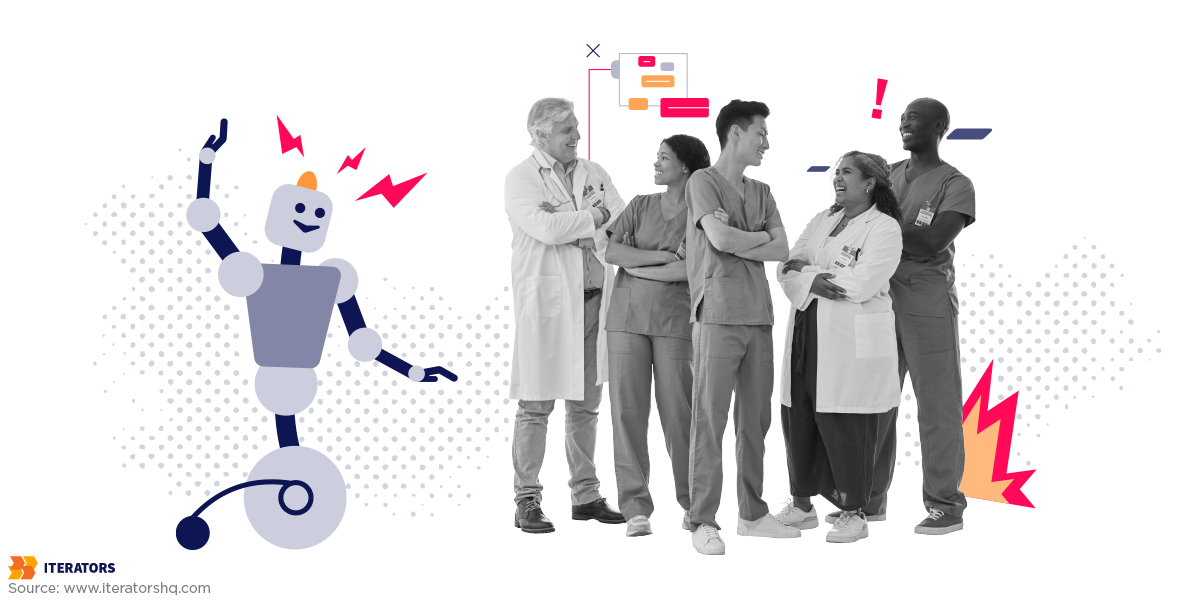
The healthcare industry is accepting AI and realizing its importance. An important driver for AI implementation is the cost savings. AI applications are estimated to reduce healthcare costs by $150 billion by 2026.
Let’s look at how AI will help healthcare professionals move forward.
1. Focus on Health Management
AI applications, particularly deep learning, help healthcare professionals and researchers find complex correlations.
However, AI assistance in treatment for clinical decision support is also welcomed. Healthcare professionals tailor medical care to individual patients using their unique differentiators, such as age, gender, lifestyle, and other biomarkers.
All these factors help to make targeted interventions.
Plus, since targeted treatments require the analysis of complex datasets, AI is a valuable tool for healthcare professionals. Its ability to provide real-time suggestions and predict patient analytics based on genomic information is revolutionary.
So, AI is exceptionally useful in clinical treatment decisions, particularly in therapy response.
2. Personalized Medicine
Dealing with chronic pain conditions can be very time-consuming, with no patient history and AI. AI tools aid in analyzing patient records and help healthcare providers determine optimal care techniques. It also helps depict hidden data trends that weren’t visible before.
This information helps to curate care plans to manage treatment and medication plans for patients.
3. Availability of Data
AI helps to gather and share data more easily. It also helps to keep a proper track of patient data and record it effectively. An example is diabetes. Studies show that about 37.3 million US residents have diabetes.
With AI, these patients can use monitoring devices to get real-time data for their glucose levels and keep track of the changes. AI algorithms also gather this information, evaluate it, and provide actionable outcomes. This data helps healthcare professionals to tailor treatment plans and manage diseases better.
Future Trends in AI
While algorithms like NLP are already being used in healthcare, they’ll become increasingly important to
- Improve patient participation in their own care
- Promote clinician productivity and care quality
- Personalize medical treatments using analytics.
AI is already working to develop precision medicine. Its contribution to early detection, diagnostic, and treatment recommendations for different diseases is also improving. Ultimately, it will take hold of this domain, too.
Given how AI is working to analyze images at an exponential rate, it’s very likely that pathology and radiology images will be examined wholly by a machine.
Speech-to-text recognition is already being used to store clinical notes, and its use will also increase. The challenge isn’t their capability but ensuring their adoption in daily clinical practice.
For widespread adoption, these AI-based software development solutions have to be approved by regulators, standardized to work in a similar way across different healthcare centers, embedded with EHR systems, taught to healthcare professionals, and maintained over time.
These challenges will be overcome, but we are many years away from seeing an extensive adoption of these AI systems.
The Takeaway
From computer-aided detection (CAD systems for diagnosis) to patient self-care, AI is already at work to streamline healthcare operations, improve efficiency, and reduce overall costs. However, advancements in AI in healthcare will not happen overnight.
Current AL development projects for healthcare have to be consistently improved to come to an acceptable level. This requires intensive product management and extensive research for each AI solution.
With that said, it takes a bit of creativity to tackle these and integrate the use of AI to deliver more streamlined healthcare solutions to all.