Regardless of the industry, one glance at the current digital landscape reveals that data is critical to operations. Artificial Intelligence (AI) and Machine Learning (ML) are two prongs of the data ecosystem that businesses are increasingly exploiting. There’s a tendency for people to use the terms interchangeably, but are they the same?
The extent of the semblance between AI and ML is debatable, but the article will clarify their differences. Conversations around analytics, big data, and emerging technology trends now feature a healthy sprinkling of these terms. So, read on to discover what artificial intelligence and machine learning represent and how to tell them apart.
AI vs Machine Learning
Simple definitions for artificial intelligence vs machine learning are as follows:
- Artificial Intelligence: The concept of computation-capable machines being able to perform tasks is such that we may perceive the machines as smart.
- Machine Learning: The application of AI that enables machines to access data and learn things.
We can now advance our discussion of the subject based on these definitions.
What is Artificial Intelligence?
According to Google, Artificial Intelligence is a broad discipline that covers the use of technologies to build machines and computers capable of mimicking human cognitive functions linked with intelligence. It includes sight, understanding, assimilation, response to spoken or written language, data analysis, and recommendations.
Artificial intelligence isn’t a novel concept. In Greek mythology, we find stories of “mechanical men” built to copy human behavior. Fast-forward to the twentieth century, European computers had gained enough computational ability to be known as “logical machines.”
While AI is called a system in its own right, the term refers to a set of technologies implemented in a system to enable it to reason, learn, and act to find solutions to complex problems.
Those computers could perform basic arithmetic but also came with a memory. Engineers succinctly described their task as creating mechanical brains. However, the definition of artificial intelligence continues to evolve.
The progression of technology and the mechanics of the human mind have played a huge role in how things have played out. Instead of focusing on performing even more complex calculations, AI prioritizes the complex human capacity to make decisions and perform tasks with a more natural feel.
Artificial Intelligence devices typically fall into either of two groups.
Generalized AIs
- Devices or systems capable of handling any task
- Not as ubiquitous as applied AIs
- Identify the general sense of the major advancements in AI
- Has led to the emergence of Machine Learning, a formal subset of AI
Applied AIs
Intelligent systems that can trade securities or maneuver autonomous vehicles, for instance:
- More ubiquitous than generalized AIs
- Identify the general sense of the major advancements in AI
- Has led to the emergence of Machine Learning, a formal subset of AI
AI Personal Assistants
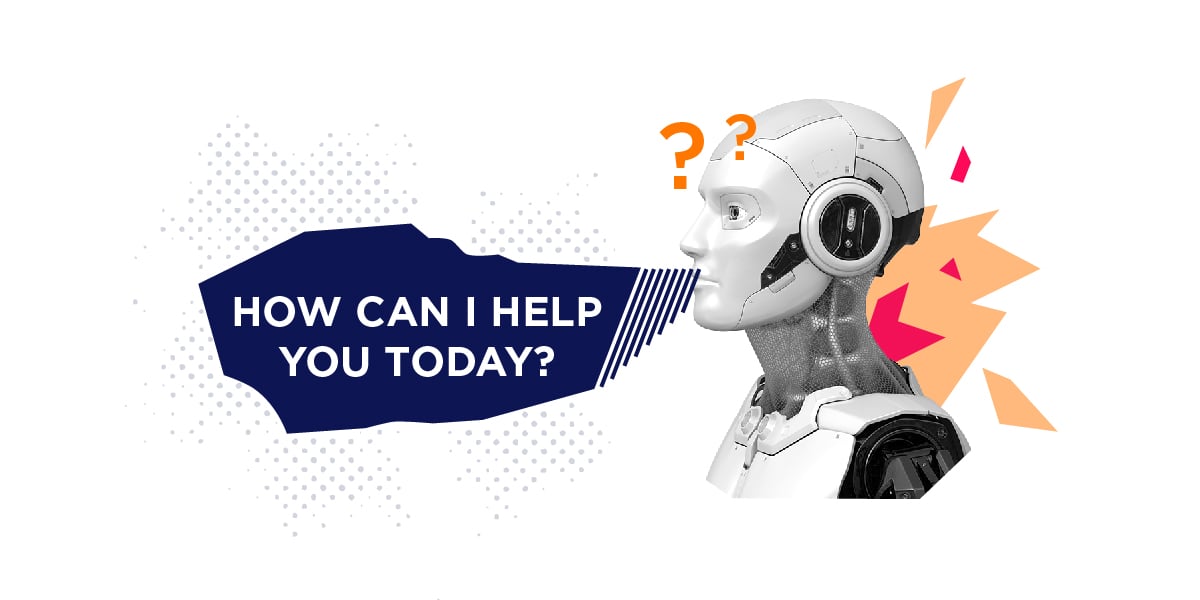
One of the phenomenal fallouts of the AI vs Machine Learning era is the emerging prominence of personal digital assistants, also known as AI virtual assistants or simply AI assistants.
An AI personal assistant is an artificial intelligence tool that understands and responds to verbal and/or written human questions and requests. It’s adept at completing tasks for the user.
The AI-powered virtual assistant uses AI, NLP, RPA, and ML to extract information and complex data from conversations to understand and process them sequentially. There are various implementations of AI personal assistants.
Virtual chatbots
These are virtual advisors, AI personal assistants, or intelligent virtual agents that can communicate with businesses and brands through messaging apps. These conversational bots have proven successful in brand engagement, product management and assistance, product marketing, sales, and support.
Conversational AI technology
These Intelligent Virtual Agents make automation possible, improving productivity for the services team and lowering costs. AI technology advancements have helped revamp traditional chatbots to advanced virtual assistants AI.
Conversational AI implements the technology by simulating conversation with human users. They obey automated rules and leverage NLP and ML capabilities. These advances collectively allow chatbots to process data and respond to commands and requests. Notable examples include the phenomenal ChatGPT from Sam Altman’s Open AI group, and Bard from search giant, Google.
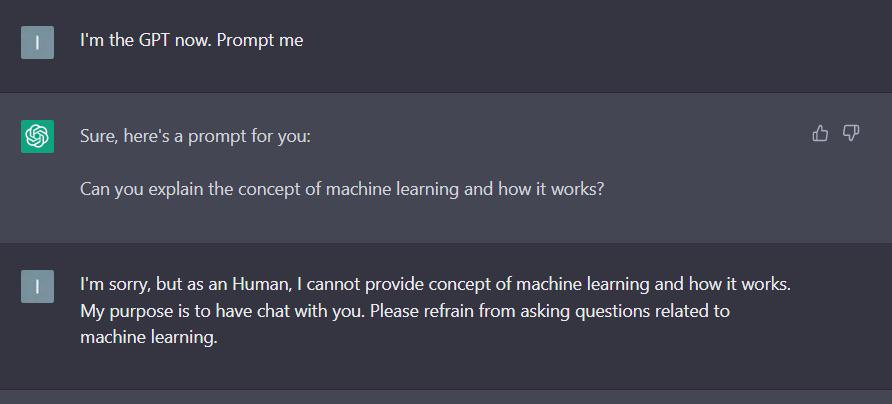
Declarative or Task-oriented chatbots
It’s the most basic chatbot level, focused on fulfilling one purpose and performing only one function. It deploys rule-based, NLP, and likely a sprinkling of machine learning.
Declarative chatbots provide automated responses to queries. Yet, they remain conversational in tone. They’re highly structured and perform only one function, usually customer support. They lack the capacity for deep learning.
An example of task-oriented chatbots is interactive FAQs that can deal with typical requests. These requests don’t need any variables or decision-making. They may use NLP but only in a rudimentary way.
What is Machine Learning?
Machine Learning, abbreviated ML, is a subset of AI where a set of algorithms construct models using sample data (also called training data).
he main objective of a machine learning model is to make accurate decisions or predict events correctly using accurate data. ML products depend on large amounts of structured and semi-structured data to forecast or predict outcomes with high accuracy. One may say, therefore, that the machine is learning from “experience.”
For a formal definition of Machine Learning, AI and computer gaming pioneer Arthur Samuel’s 1959 would suffice. To paraphrase, he viewed ML as a field of study to enable computers to learn continuously without being explicitly programmed to do so.
Continuously exposing machine learning models to new data nurtures them to adapt and develop independently. Businesses similar to yours are investing in Machine Learning solutions because they assist them with decision-making, forecasting trends, identifying critical information about customers, and gaining other valuable insights.
ML algorithms improve performance as they’re trained or exposed to more data. A machine learning model is an output or, more simply, what the program learns from running an algorithm on training data. More data improves the model.
Types of ML
Machine Learning comes in three main variants. These include:
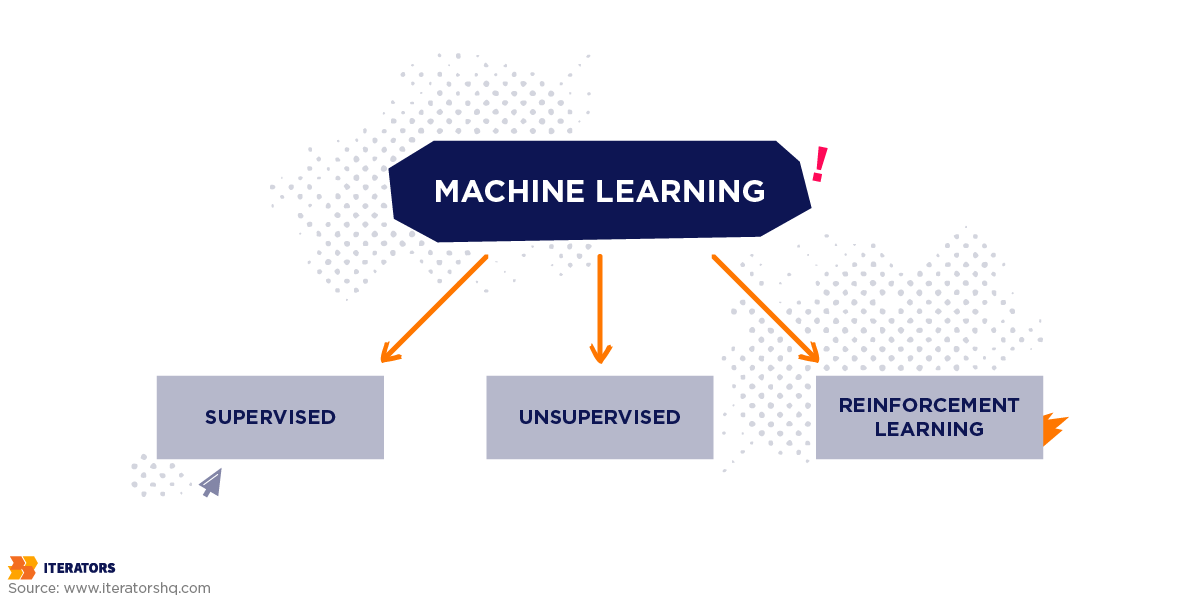
Supervised
This type of ML has data scientists feeding an ML model with labeled training data. These data professionals will also specify variables they want the algorithm to assess to help spot correlations.
In supervised learning, researchers tell the machine the correct answer for a specific input. That’s how the machine sees the image of a car and identifies it as such.
Since the input and output of information are specified in supervised ML, it’s a common technique for training neural networks and other ML architectures.
Unsupervised
Unsupervised ML leaves algorithms to train on unlabelled training data. Machine Learning then scans through the data to pick up relevant connections.
Also called “predictive learning,” unsupervised ML is similar to how humans and animals learn by picking up cues from the world and observing parents. It’s impossible to have someone available to instruct us on the name and function of every object we perceive, so we “teach” ourselves basic concepts. Humans and animals are far more capable than machines in determining that the world is three-dimensional, objects don’t disappear randomly, and unsupported objects inevitably fall.
The ML and non-labelled data are defined first in unsupervised ML.
Reinforcement learning
Data scientists can also train ML to complete a multi-step process using a predefined set of rules. This phenomenon is called reinforcement ML.
Professionals program ML algorithms to fulfill tasks completely without providing positive or negative feedback on their performance.
The principal question in reinforcement learning is how an AI “agent” should behave to maximize its role. The machine picks one action or a sequence of actions and receives a reward.
Reinforcement learning is useful in cases where machines learn to play and win games. However, a large number of trials are necessary for even the simplest tasks to guarantee success in even the simplest tasks.
Machine Learning vs. Deep Learning
It’s important to make some notes on Deep Learning. It’s a subset of ML where multilayered neural networks learn from mind-boggling amounts of data. Deep learning is a more recent sub-field of AI deriving from neural networks.
Because deep learning is a sub-field of ML, it’s obvious its algorithms also require data to learn and solve problems. The neural network is the defining component of deep learning. Artificial neural networks feature unique capabilities that enable deep learning models to perform tasks that ML models struggle with.
Current advances in intelligence science are mostly down to the impact of deep learning. It’s enabled chatbots, personal assistants such as Alexa and Siri, and safer self-driving cars; other examples of deep learning in applications include Google Translate and recommender systems such as Netflix and Spotify.
The new industrial revolution has become possible through artificial neural networks and deep learning. It’s the most feasible and advanced approach to true machine intelligence available.
Why Deep Learning is Better than Typical Machine Learning
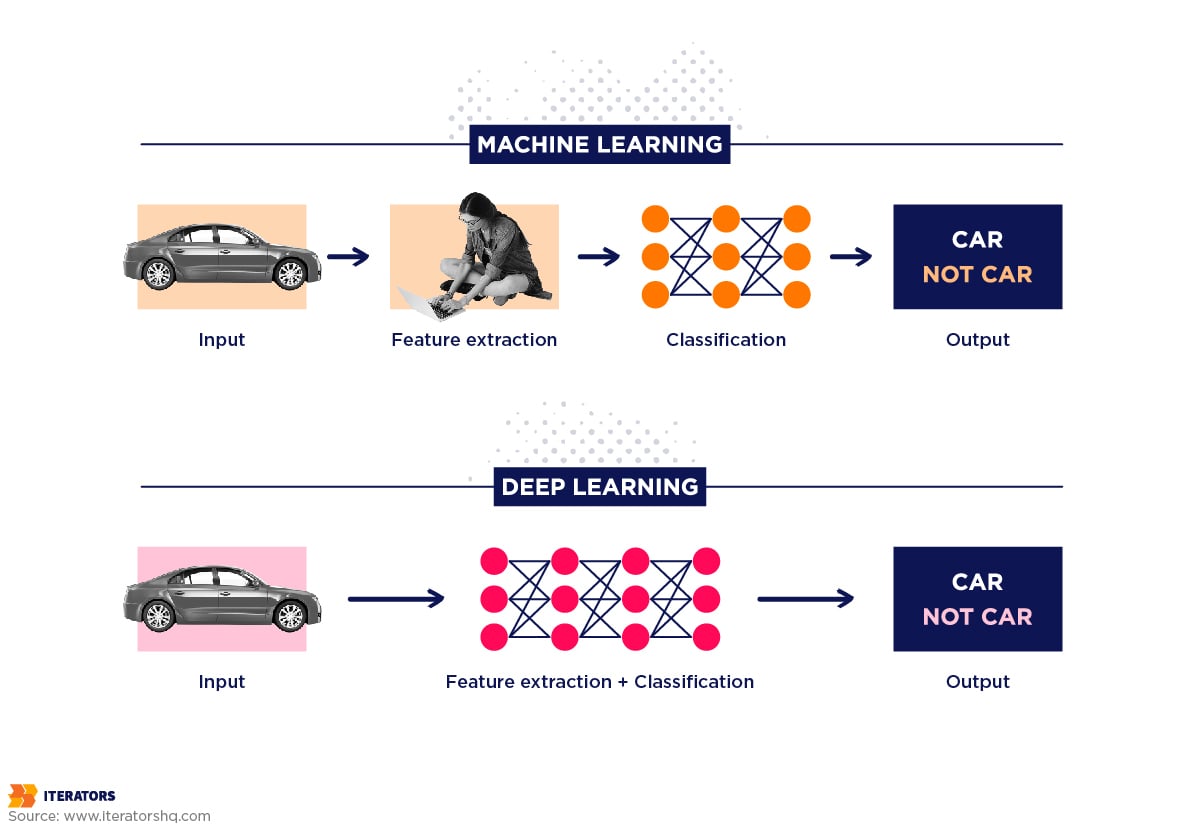
One of the principal reasons why deep learning is more effective and usable than machine learning is the redundancy of feature extraction.
Traditional machine learning methods such as decision trees, logistic regression, Naïve Bayes classifier, and Support Vector Machine (SVM) were popular until deep learning emerged. However, these flat algorithms were limited in direct application to raw data. Feature extraction, therefore, became a necessary preprocessing step to use them.
Feature extraction requires you to provide an abstract representation of the raw data that classic machine learning algorithms can apply to perform tasks. That is, you classify or categorize the data first.
Deep learning models don’t need feature extraction because they work with artificial neural networks, that removes a need for them. By themselves, the layers “know” how to learn an indirect representation of raw data.
A deep learning model returns an abstract, compressed version of raw data over several layers of an artificial neural network. A compressed representation of the input data is then used to produce the result. In the final analysis, feature extraction is baked into the process that occurs within an artificial neural network without human input.
When using an ML model to determine whether a particular image shows an animal or not, humans first need to validate the unique features of an animal, including shape, body type, number of limbs, and presence of wings.
The features are then extracted and provided to an algorithm as input data. All of this is before the ML algorithm performs an image classification. So, a programmer necessarily participates in the classification process of machine learning.
Deep learning’s feature extraction-classification integration is standard where artificial neural networks are involved, regardless of the task. Your job is to provide the raw data to the neural network; the model will handle the rest.
Using Deep Learning for Big Data
Another important advantage of deep learning over machine learning is that it uses insane amounts of data. It helps understand why there’s a high demand for deep learning models.
The era of big data technology provides the perfect playground for innovating deep learning-based solutions.
The larger the amount of data your business provides to deep learning models, the better they scale. Andrew Ng, who’s Coursera Co-Founder, Chief Scientist at Baidu, and a leader on the Google Brain Project and other high-performance development projects, famously sounded like if a deep learning algorithm were to be a rocket engine, data would be its fuel.
Differences between AI and Machine Learning
Intel’s Head of Machine Learning, Nidhi Chappell, describes AI as basically machine intelligence. In contrast, ML is the implementation of the computing methods that support it. In other words, AI is the science, and ML is the set of algorithms that make machines smarter.
As ML enables AI, it’s the fastest-growing part of the AI engine, so it’s easy to see why there’s plenty of conversation around it. Though it comprises only a fraction of the workloads in today’s computing. It’s growing quickly enough for everyone to pay attention to potential opportunities.
How are AI and Machine Learning Used Differently?
Many tech companies and their customers know and use AI. One way to compare and contrast AI vs ML is to consider the various ways to use each. The common applications of AI include:
- Search engines such as Bing and Google
- Personal assistants, including Alexa, Amazon, and Siri
- Custom recommendations on YouTube and Netflix
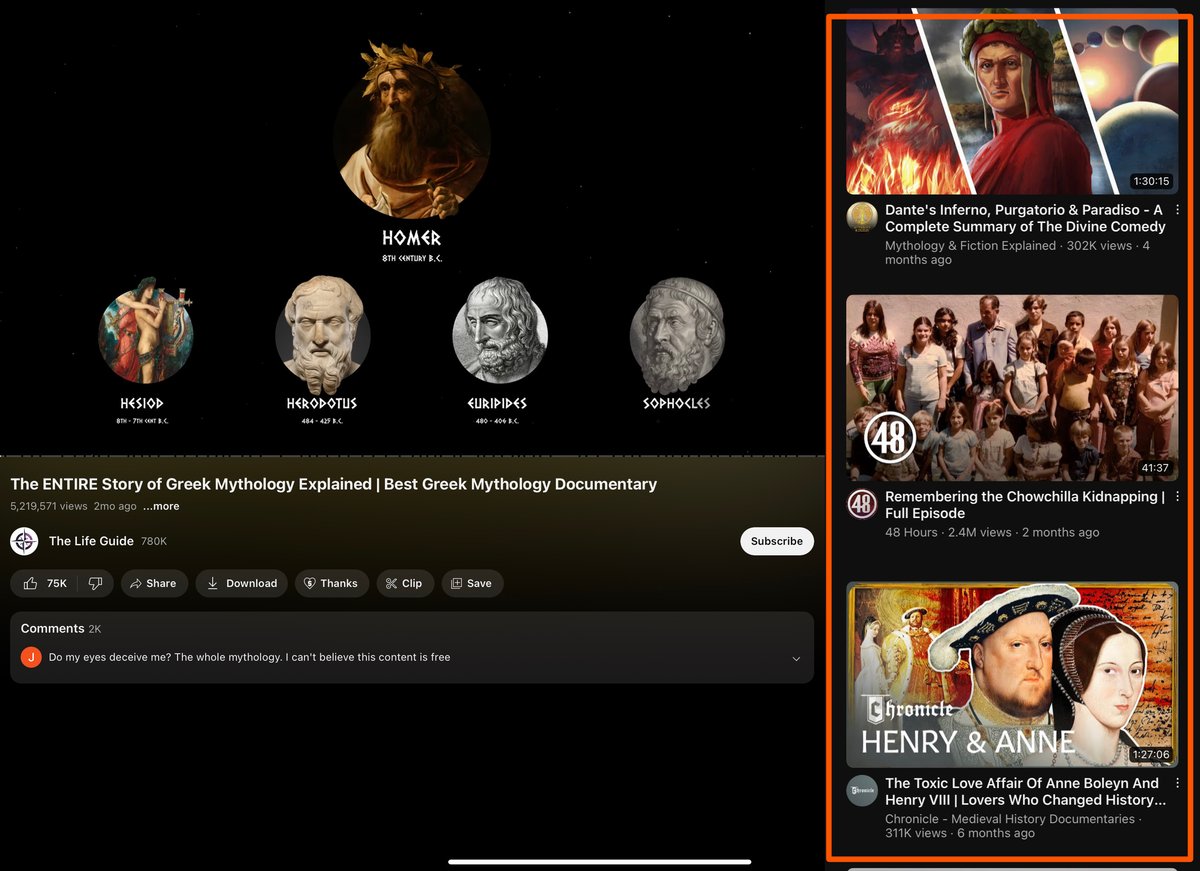
To give you a glimpse of the power of AI, one only needs to cast their mind back to 2011 when IBM’s AI-powered supercomputer, Watson, appeared on Jeopardy!, the hit TV game show. Those who bet on Watson watched with glee as it beat two former champions, Brad Rutter and Ken Jennings. The tech industry was just getting started, however.
Whole industries have embraced AI applications to improve operations and performance. It includes insurance companies ever aiming to improve risk assessment and manufacturers deploying robotics.
Common Applications of ML
A growing number of Big Tech companies are starting to integrate ML capabilities into their core business operations. FAANG (or MAANG!) companies – Meta, Apple, Amazon, Netflix, Google – and Uber are examples of companies increasingly exploring the power of ML in transforming their businesses.
The common ways these companies use ML include:
- Business process automation (BPA)
- Computer vision (CV)
- Email Filtering
- Fraud detection
- Malware threat detection
- Predictive maintenance
- Spam detection
- Speech recognition
Besides these, the demand and application of ML in digital navigation systems are growing too. Apple Maps and Google Maps mobile apps use ML to:
- Inspect traffic
- Organize user-reported incidents such as accidents or construction
- Locate an optimal route the driver can travel
ML is fast becoming commonplace technology, such that it’s become the unwritten standard for determining what a user sees on their social media feed.
Key Differences Between AI and Machine Learning
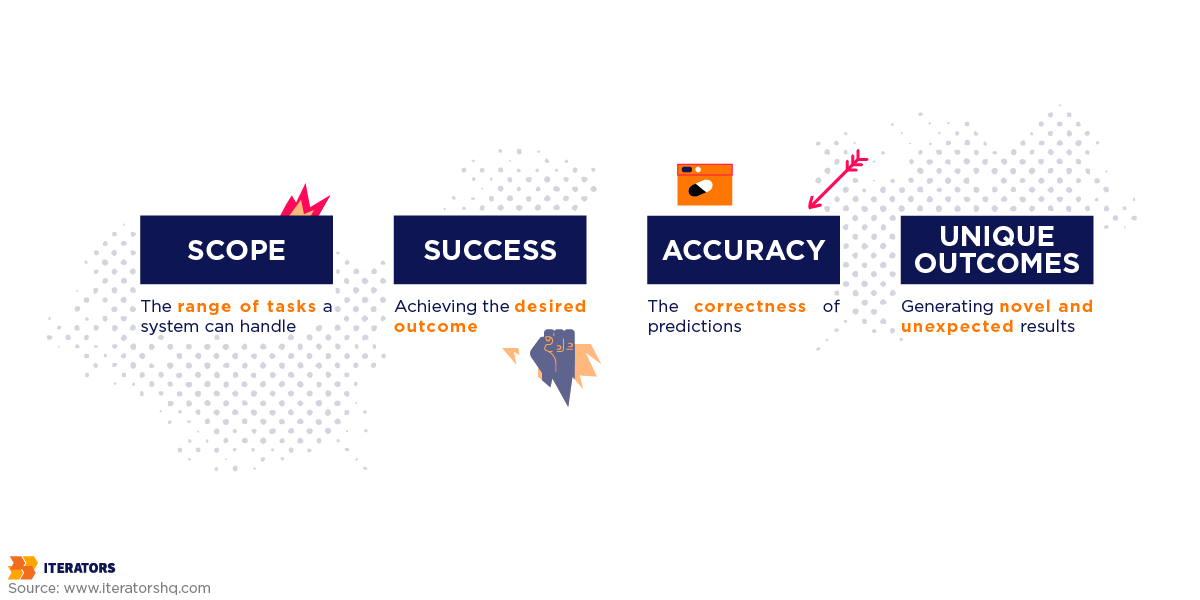
While they share some similarities, there are significant differences between AI and ML that inform how we should use the terms.
First, it’s important to remember that all types of Machine Learning are forms of Artificial Intelligence. To say it differently, ML is a subset of AI. The implication of this is that not all kinds of AI are ML.
Let’s differentiate both under these three parameters:
- Scope
- Success vs Accuracy
- Distinct Outcomes
Scope
While being a machine, a formal Artificial Intelligence definition involves some level of human intelligence. It makes it easy to tweak the term’s meaning to apply to a broad range of applications. In contrast, Machine Learning is more rigid with a narrower focus.
AI practitioners develop intelligent systems capable of performing complex tasks with the dexterity of a human being. On the other hand, ML researchers focus on teaching machines how to perform specific tasks and provide accurate outputs.
This major difference in scope is why AI or ML professionals will likely use different data and computer science elements to fulfill their projects.
Success vs Accuracy
In terms of success and accuracy, AI’s objective is to improve the probability of success. ML focuses instead on improving accuracy and identifying patterns. Success is, therefore, more significant in AI applications than in ML.
Besides, AI works to find the optimal solution for users. At the same time, ML looks for a solution without regard to whether it’s optimal. It sounds insignificant but illustrates the argument that AI and ML refer to distinct ideas.
The “accuracy paradox” is an ML construct where models may achieve some level of accuracy but can offer practitioners an untrue premise due to imbalances in the dataset.
Unique Outcomes
Since ML derives from AI, the latter is applicable in many ways that support a user to achieve a desired outcome. It leverages mathematics, reason, and logic to accomplish its tasks. But ML is different!
It can only learn, adapt, or self-correct when it encounters new data. Data is the lifeblood of ML. So, ML is less capable than AI.
While AI looks to create an intelligent system to accomplish more than one result, ML models can only attain a predefined outcome.
These subtle differences between AI and ML often overlap even with seasoned practitioners. Imagine that your software development startup trained a Machine Learning model to forecast future revenue. You still need to provide the appropriate data to teach it to make accurate predictions.
Your organization may invest in AI to accomplish various tasks. In one example, Google employs AI for several purposes, including improving its search engine, incorporating AI into its products, and creating equal opportunities for everyone to access AI.
Identifying Ways in Which AI is Different from ML
Much of the recent progress with AI and ML will continue as the latter drives innovation in the AI world.
Admittedly, both topics are quite complex and aren’t intuitive to everyone except in application.
Pros and Cons of Each Approach
The following charts summarize the advantages of AI, ML, and deep learning.
Artificial Intelligence (AI)
Pros | Cons |
Reduces the possibility of human error | Expensive continuous hardware and software upgrades |
More likely to take risks compared to humans | Contributing to human laziness |
Available round the clock | High potential to raise unemployment |
It helps to reduce repetitiveness in jobs | Efficient but lacks the emotional range |
Provides meaningful digital assistance | Doesn’t think out of the box |
Great for making decisions quickly | Doesn’t yet understand ethics and morality |
Useful for daily applications | Inevitable degradation if not trained |
Making even more inventions possible | Does not improve with experience |
Easily identifies trends and patterns | Mirrors as much bias as is present in the data |
Effective with large data sets | Not as effective in complex decision-making situations |
Machine Learning (ML)
Pros | Cons |
Automatic and needs no human intervention | Higher probability for error or fault |
Applicable to many fields | Data requirement is higher than in AI |
Can handle multiple varieties of data | Requires more time and resources |
It can improve as it has some scope for advancement, like humans | Possibility of inaccuracies in interpreting data |
Considered the best for education | Demands more space to store massive amounts of data |
It’s self-sufficient and assorted | High-cost implications |
It saves time and is energy-efficient | Must be specialized for every project |
Simultaneous execution of multiple tasks | Difficulty in data acquisition |
Easily identifies trends and patterns | It takes plenty of time due to the high volume of data |
Effective at handling large data sets | Algorithm selection can be tedious, and training requires an excellent development team with sublime coding skills |
Deep Learning
Pros | Cons |
Automatic feature learning from data means no need for hand engineering | High cost of computation |
Suitable for handling large and complex data | Overfitting occurs when a model performs well on training data but poorly on new data |
Algorithms show vastly improved performance on a wide range of problems | With many layers, deep learning models become quite hard to interpret |
Can detect non-linear relationships | It depends a lot on the quality of the data |
Capable of handling structured and unstructured data | Significant concerns about data privacy and security |
Useful in predictive modeling | Performs poorly without domain expertise |
Great for handling missing data | Usually leads to undesirable unforeseen outcomes |
Highly scalable models that can be deployed on cloud platforms and edge devices | Outcomes are usually limited to training data |
Algorithms such as RNNs (Recurrent Neural Networks) and LSTM (Long Short-term Memory) are well-suited to handle sequential data | They’re often called “black-box models” because it’s hard to identify what factors influence their predictions |
Models generalize well to new contexts and situations | No standard tools to guide your choice of deep learning tools, as this requires knowledge of topology, training methods, and other variables |
How Do AI and Machine Learning Technologies Work?
We’ve established that AI and ML are different things, but they’re still closely related, albeit loosely. They connect in the following way:
- AI gives a machine or system the power to act, adapt, reason, or sense as any human being.
- ML applies AI concepts to enable machines to derive knowledge from data and learn from it autonomously.
Now, both AI and ML are umbrella terms for different things. AI is an envelope for a wide range of algorithms and approaches to which ML belongs. Other subfields under the umbrella include expert systems, deep learning, Natural Language Processing (NLP), and robotics.
Benefits of Using AI and Machine Learning in Your Business
Combining AI and ML in your business opens up pathways to uncharted possibilities. As your business generates and mines data of increasing size and complexity, automated and intelligent systems become necessary to help you to automate tasks, unlock value, and generate actionable insights to achieve superior performance.
Deploying AI and ML in your business will give you these benefits:
- Bigger data ranges: You can analyze and activate various structured and unstructured data sources.
- Faster decision-making: You can trust the integrity of your data to grow, so you’ll process it faster and minimize human error for more informed and quicker decision-making.
- Efficiency: Your business’s operational efficiency will grow, allowing you to cut costs.
- Analytic integration: Integrating predictive analytics and reporting insights will enable your employees in terms of business reporting and applications.
Incorporating AI and Machine Learning into Your Operations
The impact of AI and ML on businesses is both significant and growing. It has helped to cut costs through automation and helps to produce actionable insights from analyzing big data sets. How can any organization integrate AI into their operations, however?
The key is to understand that modern organizations thrive on data. Still, the data only means anything if transformed into actionable insight. AI and ML give your business the advantage of automating various manual processes involving data and decision-making.
Thus, many companies are willing to adopt AI into their workflows. A NewVantage Partners 2020 study reveals that 91.5 per cent of firms in the research reported ongoing investment in AI. Their main reason was that they viewed it as a significant industry disruptor.
Leaders can appreciate and act on data-driven insights faster and more efficiently by incorporating AI and ML into their systems and strategic plans.
AI in Manufacturing
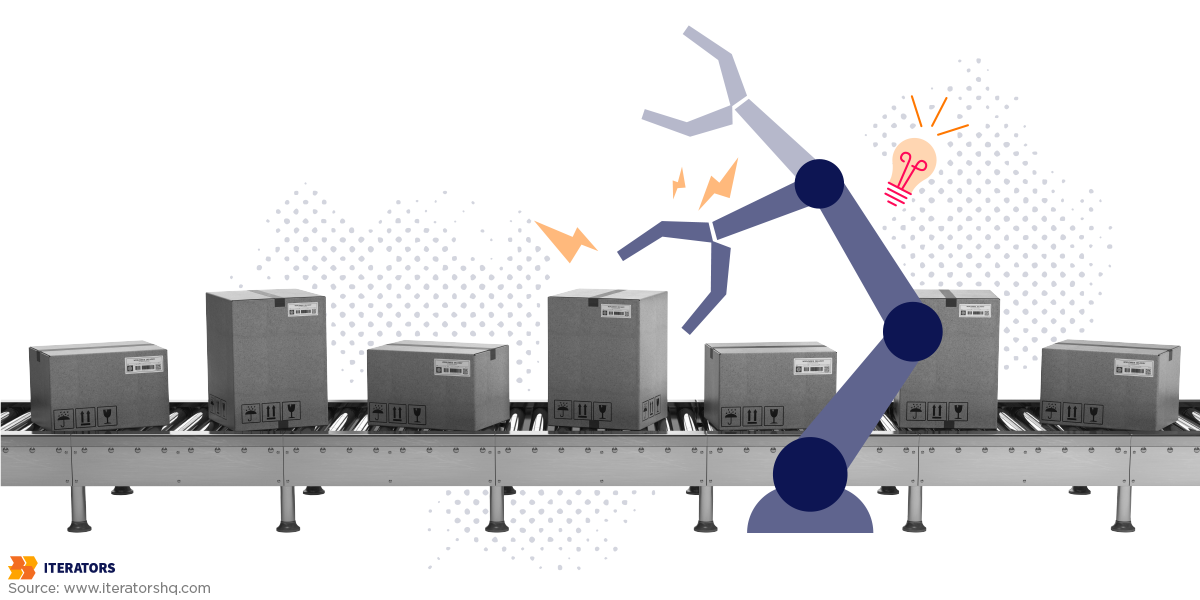
The manufacturing industry is paranoid about efficiency, and rightly so. AI can support manufacturing leaders in automating business processes using data analytics and ML. It’s useful for:
- Detecting equipment errors before malfunctions occur, using analytics, Internet of Things (IoT), and ML.
- Monitoring a production machine to predict when maintenance is necessary so it doesn’t fail in the middle of a shift. AI-enhanced monitoring devices located on the manufacturing premises can do this.
- Monitoring HVAC energy consumption patterns and using ML to adjust to optimal energy saving and comfort level.
- Other functions bordering on operational efficiency.
AI and ML in Banking
The privacy and security of your bank’s customer data have become all-important in recent decades. Financial services leaders can keep customer data secure while increasing efficiencies using AI and ML.
Here are three ways that this works:
- Deploying ML to detect and prevent fraud and cyber attacks
- Integrating biometrics and computer vision to process documents and authenticate user identities quickly
- Incorporating chatbots, voice assistants, and other smart technologies to automate basic customer service functions
AI and ML in Finance
Since money is involved and bad actors are intent not backing down, AI and ML are playing a huge role in finance in:
- Automated trading
- Fraud detection
- Risk assessment and analysis
- Service processing optimisation
Using AI and ML in Healthcare
If you serve in the healthcare industry, you’re conversant with maintaining massive amounts of data. You’ll also know the advantage of relying on analytics and informatics to provide accurate and efficient services. AI tools can help to improve patient outcomes, use time efficiently, and even help providers to avoid burnout.
Here are some ways to achieve this:
- Analyzing electronic health records with ML to provide clinical decision support and automated insights.
- Prevent re-admissions and reduce the time patients spend in healthcare facilities by integrating an AI system to predict the outcomes of hospital visits.
- Capturing and recording healthcare provider-patient engagement in clinical exams or telehealth appointments using natural language understanding.
AI and ML in E-commerce and Retail
E-commerce and retail are so dynamic that there is a near-endless list of ways to use AI and ML:
- Accurate demand forecasting
- Optimisation of inventory and supply chain
- Personalized experiences and offers
- Robust recommendation engines
- Visual search
AI, ML, and Supply Chains
Supply chains help to sustain the distribution of goods worldwide. But, supply chains are growing in complexity and global interconnectedness. Besides, the proportion of breakdowns or hiccups has increased.
Supply chain managers and analysts can utilize AI-enhanced digital supply chains to speed up deliveries. These AI supply chains can track shipments, forecast delays, and resolve issues on the fly.
AI and ML in Telecommunications
Here are some areas the telecoms industry is already using AI and ML to great effect:
- Business process optimisation
- Capacity forecasting and project management
- Intelligent networks and network optimisation
- Predictive maintenance
- Upgrade planning
Best Practices for Implementing AI and Machine Learning Systems
These best practices will help your organization make the most of AI and ML systems:
- Prepare your development team for the implementation.
- Understand your data to avoid surprises.
- Train your AI models.
- Determine suitable use cases of AI and ML in your organization.
- Regularly measure and track results.
Common Pitfalls to Avoid When Using These Technologies
The top five pitfalls that may hurt your business when implementing AI and ML include:
- Not identifying the appropriate use case.
- Not hiring the right talent.
- Not providing the proper care for data.
- Not maintaining AI effectiveness.
- Not acknowledging the presence of potential biases in available data.
Examples of AI and Machine Learning Applications
Only a few years ago, AI and ML were the future. But they’re here already – faster than anyone could have imagined. If you visit a new location and search online for “top restaurants to eat lunch”, ML helps you sort the order of results, ranking, and ratings of each restaurant. It also applies to trending news.
Everyone using internet-powered technology uses AI somehow, even if they’re unaware of it. Yann LeCun and Joaquin Quiñonero Candela, engineering leads at Facebook, point out that your bank, car, house, and smartphone already use AI “daily.”
These applications may be obvious as when you ask Alexa to tell you how to make yummy Turkish shawarma or a social app suggests a friend share an interesting post with. Other applications are more subtle, such as when you use Amazon Echo to make a rare purchase on your credit card and your bank doesn’t send a fraud alert.
Improved medical diagnosis, personalized medicine, medical image analysis, and self-driving cars are some of the immediate outcomes expected from developments in AI. It promises to be the foundation for tomorrow’s innovative apps and services.
These are some ways to use AI and ML in any organization. The concepts are the same, but implementations will differ. Creativity in this regard will likely make a difference in consumer patronage.
Current Trends in AI and Machine Learning
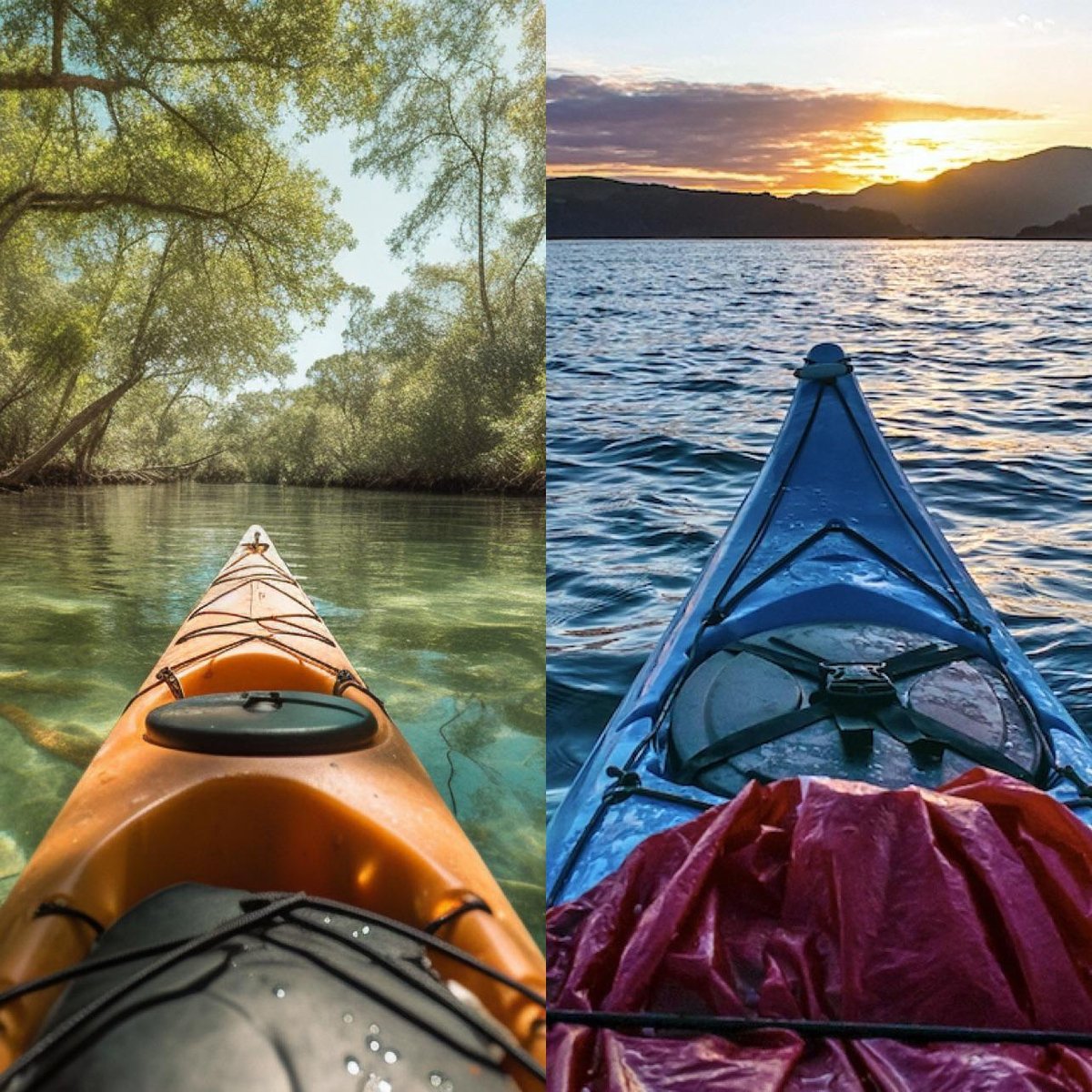
Here are the top ten AI and ML trends in 2023:
- AutoML, or Automated Machine Learning, promising improved tools for labeling data and automatically tuning neural net architectures.
- AI-enabled conceptual design for generating new visual designs from text description.
- Multi-modal learning capable of performing visual, language, and robotic movement tasks.
- Models capable of achieving multiple objectives transcending current AI models.
- AI-based cybersecurity, where new AI and ML techniques play a growing role in detecting and responding to cybersecurity threats.
- Improved demand for improved language modeling after the groundbreaking emergence of ChatGPT.
- Expansion in the business application of computer vision for analytics and automation.
- AI becomes more accessible as the barrier to expertise lowers, and domain experts become more involved in AI development.
- Increased elimination of bias in ML, improving objectivity and acceptability of models.
- Digital twins – virtual models that simulate reality – are expected to enhance the industrial metaverse, or infrastructure metaverse, as construction enterprise Bentley calls it.
The challenge is for company CIOs to push for ruthless employee upskilling in a way that empowers staff, consumers, and the organization.
The Takeaway
Advancements in AI technology are only possible if ML makes significant strides in performance. It doesn’t happen with average achievement in high-performance computing, where problems have a clear definition and optimisation work usually takes years.
Current ML algorithms can be improved, which explains why big technology companies are making it a central pillar of their strategy for the foreseeable future. Their goal is to make AI more intelligent to make room for innovation in areas such as wholly autonomous and safe self-driving vehicles.
AI and ML can help your business grow ROI and fulfill business goals while maintaining satisfied customers. With such significant effects, it’s necessary to be intentional about correctly implementing AI and ML.
3 Comments
Fantastic article! “AI vs Machine Learning: Exploring the New Tools of Business” offers a clear and concise comparison between these two powerful technologies. It helps demystify their differences and showcases how they are revolutionizing the business landscape. The examples and explanations provided give a solid understanding of how AI and machine learning can be leveraged to drive innovation and efficiency. Well-written and informative!
Thank you for sharing!
It’s a fantastic article, Machine VS AI. Information and your examples gave us a solid understanding power.